Tutorials
Abstract
We have witnessed a remarkable progress of deep learning in medical image analysis over the past few years. Specifically, deep learning models are playing an essential role in the medical imaging field, including computer-assisted diagnosis, image segmentation, image registration, image fusion, image-guided therapy, image annotation, and image database retrieval. With advances in medical imaging, new imaging modalities and methodologies, and new deep learning algorithms/applications, come to the stage for medical imaging. In recent years, a lot of deep learning models have successfully applied to medical image analysis tasks. Despite their success, the challenge remains that current deep learning methods are data-hungry in nature, which usually require large-scale, well-annotated, few noisy labels or delineation. In medical image analysis field, it is known that data and annotations are expensive to acquire. In this meaning, annotation-efficient deep learning models, e.g., weakly supervised learning, transfer learning, self-supervised learning, have aroused considerable attention for medical image analysis. This tutorial will introduce several recent progress in annotation-efficient deep learning models and their application in medical image analysis.
Keywords
Weakly-supervised learning; Self-supervised learning; Transfer learning; Medical image segmentation; Computer-aided diagnosis; Noisy label
Instructors
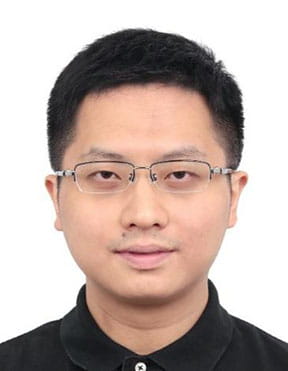
Yinghuan Shi , Nanjing University, China. (syh [AT] nju.edu.cn)
Yinghuan Shi is currently an Associate Professor in the State Key Laboratory for Novel Software Technology at Nanjing University, China. He is also Principal Researcher of Medical Imaging Center in the National Institute of Healthcare Data Science at Nanjing University, China. He obtained his both Ph.D and BS. Degrees from Nanjing University in 2013 and 2007, respectively. Dr. Shi has published over 70 papers in related conference or journals, e.g., TPAMI, TIP, TMI, CVPR and MICCAI. Dr. Shi was elected as Young Elite Scientists Sponsorship Program by CAST. Also, he was awarded as WuWenJun AI Excellent Young Scientist in 2019, ACM Nanjing Rising Star in 2017, and Jiangsu Computer Federation Excellent Young Scientist Award in 2017. Currently, Dr. Shi is broadly interested in medical image analysis, clinical data mining, and also including the other related topics in image processing, computer vision, and machine learning. In particular, the main goal of his research is to develop the machine learning-based algorithms for the data analysis problems in medical imaging and clinical data. The difficulties in medical data, e.g., complicated connection, unpredictable noise, limited amount and insufficient labeling, pose the considerable challenges for the data analysis. He wishes to develop the effective and efficient methods to tackle these challenges. Also, these proposed methods are used to guide the development of the real systems in the clinical scenario.
Abstract
Internet of Things (IoT) is the network of physical objects or “things” embedded with electronics, software, sensors, and network connectivity. It enables the objects to collect, share, and analyze data. The IoT has become an integral part of our daily lives through applications such as public safety, intelligent tracking in transportation, industrial wireless automation, personal health monitoring, and health care for the aged community. IoT is one of the latest technologies that will change our lifestyle in the coming years. Experts estimate that as of now, there are 23 billion connected devices, and by 2025 it would reach 30 billion devices. This tutorial aims to introduce the design and implementation of IoT systems. The foundations of IoT to build a project will be discussed throughout real applications. Challenges and constraints for future research in IoT will be discussed. In addition, research opportunities and collaboration will be offered for the attendees.
Keywords
Big data; Cloud computing; Internet of things; Sensors
Instructors
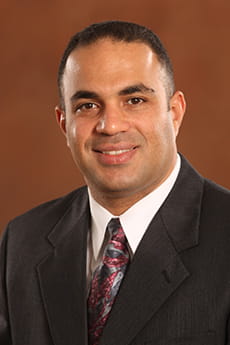
Ahmed Abdelgawad , Central Michigan University, USA. (abdel1a [AT] cmich.edu)
Dr. Ahmed Abdelgawad received his M.S. and a Ph.D. degree in Computer Engineering from University of Louisiana at Lafayette in 2007 and 2011 and subsequently joined IBM as a Design Aids & Automation Engineering Professional at Semiconductor Research and Development Center. In Fall 2012 he joined Central Michigan University as a Computer Engineering Assistant Professor. In Fall 2017, Dr. Abdelgawad was early promoted as a Computer Engineering Associate Professor. He is a senior member of IEEE. His area of expertise is distributed computing for Wireless Sensor Network (WSN), Internet of Things (IoT), Structural Health Monitoring (SHM), data fusion techniques for WSN, low power embedded system, video processing, digital signal processing, Robotics, RFID, Localization, VLSI, and FPGA design. He has published two books and more than 78 articles in related journals and conferences. Dr. Abdelgawad served as a reviewer for several conferences and journals, including IEEE WF-IoT, IEEE ISCAS, IEEE SAS, IEEE IoT Journal, IEEE Communications Magazine, Springer, Elsevier, IEEE Transactions on VLSI, and IEEE Transactions on I&M. He served in the technical committees of IEEE ISCAS 2007, IEEE ISCAS 2008, and IEEE ICIP 2009 conferences. He served in the administration committee of IEEE SiPS 2011. He also served in the organizing committee of ICECS2013 and 2015. Dr. Abdelgawad was the publicity chair in North America of the IEEE WF-IoT 2016/18/19 conferences. He was the finance chair of the IEEE ICASSP 2017. He is the TPC Co-Chair of I3C'17, the TPC Co-Chair of GIoTS 2017, and the technical program chair of IEEE MWSCAS 2018. He was the keynote speaker for many international conferences and conducted many webinars. He is currently the IEEE Northeast Michigan section chair and IEEE SPS Internet of Things (IoT) SIG Member. In addition, Dr. Abdelgawad served as a PI and Co-PI for several funded grants from NSF.
Abstract
Although automatic fingerprint identification system (AFIS) has been widely used for more than 2 decades in criminal identification, background checks, border control, and many other applications, several problems still persist in current systems: (1) manual feature annotation is required for latent fingerprints, (2) searching a billion-scale database is prohibitively slow. We show how to overcome these limitations with innovations in image processing, hierarchical feature representation, and heterogeneous computation. Using these techniques, we have built a billion-scale fingerprint database that supports high-accuracy, high-throughput, and fully automated fingerprint matching, bringing revolution to the industry.
For civilian biometric applications, privacy is a major concern. A privacy-preserving biometric system should have the following properties: (1) non-invertibility, (2) revocability, (3) non-linkability. While it has been an active research topic over the last 20 years, existing solutions are still far from gaining practical acceptance. We survey existing methods and point out directions to improve the security and performance of current methods.
Contactless fingerprint acquisition is an emerging technology that is easier to use and more hygienic, and it’s attracting even more attention during the COVID-19 pandemic. Leveraging a specially designed structured light and stereo vision hybrid system, as well as innovative image processing techniques, we can capture large-area, high-quality contactless fingerprints.
Combining these innovations, we hope to build the next-generation fingerprint technology that is more accurate, more secure, and more user-friendly, seamlessly connecting the digital and physical identity, and making the world safer and more convenient.
Keywords
Big multimedia data design and creation; Multimedia security; biometric data privacy; Multimedia analysis; search and recommendation; contactless fingerprint acquisition
Instructors
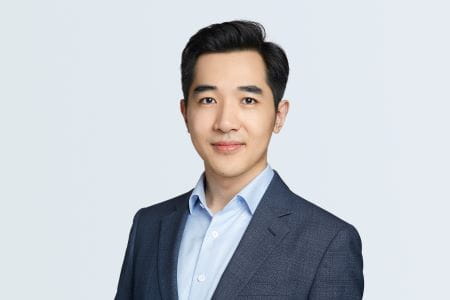
Cheng Tai , Moqi, Inc., China. (chengt [AT] moqi.ai)
Dr, Cheng Tai is the co-founder and CEO of Moqi, Inc. He oversees the development of next-generation AI products aiming to enhance the processing of unstructured data at scale. Developed an innovative product that matches biometric features at a large scale, built two billion-scale databases, and completely changed the law enforcement fingerprint and palmprint matching landscape.
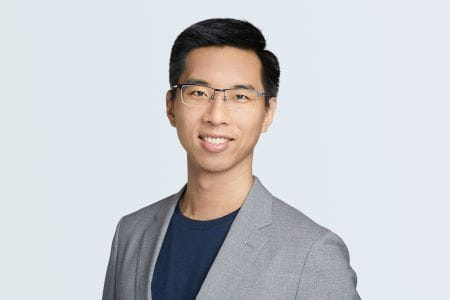
Linpeng Tang , Moqi, Inc., China. (linpengt [AT] moqi.ai)
Dr. Lingpeng Tang is the co-founder and CTO of Moqi, Inc. At Moqi, he builds innovative technology and products aiming to transform biometrics and unstructured data processing in general, including but not limited to: (1) fully automated fingerprint identification system that matches 2 billion fingerprints in seconds with high accuracy, (2) large-area, high-quality contactless fingerprint acquisition device, (3) privacy-preserving biometrics systems.
Abstract
The ubiquitous surveillance cameras are generating huge amount of videos and images. Automatic content analysis and knowledge mining techniques are thus desirable for effective storage and utilization of these data. As a research topic attracting more and more interests in both academia and industry, person Re-Identification (ReID) targets to identify the re-appearing persons from a large set of videos. It is potential to open great opportunities to address the challenging data storage problems, offering an unprecedented possibility for intelligent video processing and analysis, as well as exploring the promising applications on public security like cross camera pedestrian searching, tracking, and event detection.
The proposed tutorial aims at reviewing the latest research advances, discussing the remaining challenges in person ReID, and providing a communication platform for researchers working on or interested in this topic. It covers our latest works on person ReID, e.g., the deep model design, discriminative local and global representation learning, Generative Adversarial Networks for data augmentation and dataset transfer, unsupervised person ReID model training, as well as our viewpoints about the unsolved challenging issues in person ReID. We believe this tutorial would be helpful for researchers working on person ReID and other related topics.
Instructors
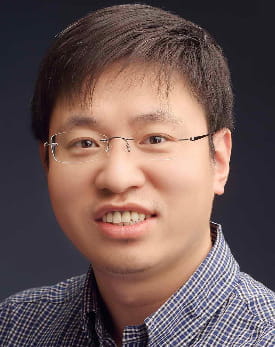
Shiliang Zhang , Peking University, China. (slzhang.jdl [AT] pku.edu.cn )
Shiliang Zhang is currently an Assistant Professor in Department of Computer Science, School of Electronic Engineering and Computer Science, Peking University. He received the Ph.D. degree in computer science from Institute of Computing Technology, Chinese Academy of Sciences in 2012. He was a Postdoctoral Scientist in NEC Labs America and a Postdoctoral Research Fellow in University of Texas at San Antonio.
Dr. Zhang’s research interests include large-scale image retrieval, fine-grained visual recognition, and person re-identification. He has author or co-authored over 80 papers in articles in journals and conferences, including the International Journal on Computer Vision, IEEE Transactions on PAMI, the IEEE Transactions on Image Processing, IEEE Transactions on Multimedia, ACM Multimedia, ICCV, CVPR, and ECCV. He was a recipient of the First Prize of Technical Invention by Ministry of Education of China, Outstanding Doctoral Dissertation Awards from the Chinese Academy of Sciences and the Chinese Computer Federation, the President Scholarship from the Chinese Academy of Sciences, the NEC Laboratories America Spot Recognition Award, and the Microsoft Research Fellowship. He was also a recipient of the Top 10% Paper Award at the IEEE MMSP 2011. His research is supported by the key project of Natural Science Foundation of China (NSFC), National Key Research and Development Program of China, Outstanding Young Scholar Fund of Beijing Natural Science Foundation, etc.
Abstract
In the recently years, screen content video including computer generated text, graphics and animations, have drawn more attention than ever, as many related applications become very popular. However, conventional video codecs are typically designed to handle the camera-captured, natural video. Screen content video on the other hand, exhibits distinct signal characteristics and varied levels of the human’s visual sensitivity to distortions. To address the need for efficient coding of such contents, a number of coding tools have been specifically developed and achieved great advances in terms of coding efficiency.
The importance of screen content applications is well addressed by the fact that all of the recently developed video coding standards have included screen content coding (SCC) features. Nevertheless, the inclusion considerations of SCC tools in these standards are quite different. Each standard typically adopts only a subset of the known tools. Further, for one particular coding tool, when adopted in more than one standard, its technical features may various quite a lot from one standard to another.
All these caused confusions to both researchers who want to further explore SCC on top of the state-of-the-art and engineers who want to choose a codec particularly suitable for their targeted products. Information of SCC technologies in general and specific tool designs in these standards are of great interest. This tutorial provides an overview and comparative study of screen content coding (SCC) technologies across a few recently developed video coding standards, namely HEVC SCC, VVC, AVS3, AV1 and EVC. In addition to the technical introduction, discussions on the performance and design/implementation complication aspects of the SCC tools are followed up, aiming to provide a detailed and comprehensive report. The overall performances of these standards are also compared in the context of SCC. The SCC tools in discussion are listed as follows:
Screen content coding specific technologies:
- Intra block copy (IBC)
- Palette mode coding (PLT)
- Transform Skip Residue Coding (TSRC)
- Block based differential pulse code modulation (BDPCM)
- Intra string copy (ISC)
- Deblocking filter (DBK)
Screen content coding related technologies:
- Integer motion vector difference (IMVD)
- Intra subblock partitioning (ISP)
- Geometrical partition blending off (GPBO)
- Adaptive Color Transform (ACT)
- Hash based motion estimation (HashME)
Instructors
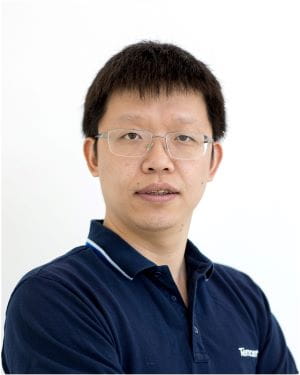
Xiaozhong Xu , Tencent Media Lab, USA. (xiaozhongxu [AT] tencent.com)
Xiaozhong Xu has been a Principal Researcher and Manager of Multimedia Standards at Tencent Media Lab, Palo Alto, CA, USA, since 2017. He was with MediaTek USA Inc., San Jose, CA, USA as a Senior Staff Engineer and Department Manager of Multimedia Technology Development, from 2013 to 2017. Prior to that, he worked for Zenverge (acquired by NXP in 2014), a semiconductor company focusing on multi-channel video transcoding ASIC design, from 2011 to 2013. He also held technical positions at Thomson Corporate Research (now Technicolor) and Mitsubishi Electric Research Laboratories. His research interest lies in the general area of multimedia, including video and image coding, processing and transmission. He has been an active participant in video coding standardization activities for over fifteen years. He has successfully contributed to various standards including H.264/AVC and its extensions, AVS1 and AVS3 (China), HEVC and its extensions, MPEG-5 EVC and the most recent H.266/VVC standard. He served as a core experiment (CE) coordinator and a key technical contributor for screen content coding developments in various video coding standards (HEVC, VVC, EVC and AVS3). Xiaozhong Xu received the B.S. and Ph.D. degrees from Tsinghua University, Beijing China in electronics engineering, and the MS degree from Polytechnic school of engineering, New York University, NY, USA, in electrical and computer engineering.
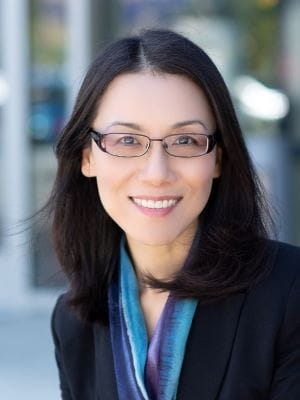
Shan Liu , Tencent Media Lab, USA. (shanl [AT] tencent.com)
Shan Liu received the B.Eng. degree in electronic engineering from Tsinghua University, the M.S. and Ph.D. degrees in electrical engineering from the University of Southern California, respectively. She is now a Tencent Distinguished Scientist and General Manager of Tencent Media Lab. She was formerly Director of Media Technology Division at MediaTek USA. She was also formerly with MERL, Sony Electronics and Sony Computer Entertainment America (now Sony Interactive Entertainment). She has been actively contributing to international standards since the last decade and served co-Editor of HEVC SCC and the emerging VVC. She has numerous technical contributions adopted into various standards, such as HEVC, VVC, OMAF, DASH and PCC, etc. She also directly contributed to and led the development effort of products which have served hundreds of millions of users. Dr. Liu holds more than 150 granted US and global patents and has published more than 80 journal and conference papers. She was in the committee of Industrial Relationship of IEEE Signal Processing Society (2014-2015) and is on the Editorial Board of IEEE Transactions on Circuits and Systems for Video Technology (2018-2021). She was the VP of Industrial Relations and Development of Asia-Pacific Signal and Information Processing Association (2016-2017) and was named APSIPA Industrial Distinguished Leader in 2018. She was appointed Vice Chair of IEEE Data Compression Standards Committee in 2019. Her research interests include audio-visual, high volume, immersive and emerging media compression, intelligence, transport, and systems.
Abstract
The tutorial discusses an open and freely available encoder implementation VVenC of the latest video coding standard VVC (Versatile Video Coding) jointly developed by ITU-T and ISO/IEC. VVC has been designed to achieve significantly improved compression capability compared to previous standards such as HEVC, and at the same time to be highly versatile for effective use in a broadened range of applications.
Some key application areas for the use of VVC particularly include ultra-high-definition video (e.g. 4K or 8K resolution), video with a high dynamic range and wide color gamut (e.g., with transfer characteristics specified in Rec. ITU-R BT.2100), and video for immersive media applications such as 360° omnidirectional video, in addition to the applications that have commonly been addressed by prior video coding standards. Important design criteria for VVC have been low computational complexity on the decoder side and friendliness for parallelization on various algorithmic levels. VVC has been finalized in July 2020 and in September 2020. Fraunhofer HHI has made an optimized VVC software encoder (VVenC) and a VVC software decoder (VVdeC) implementations publicly available on GitHub.
The tutorial details the open encoder implementation VVenC with a specific focus on the challenges and opportunities in implementing the myriad of new coding tools. This includes algorithmic optimizations for specific coding tools such as block partitioning, motion estimation as well as implementation specific optimization such as SIMD vectorization and parallelization approaches. In addition to runtime optimizations, subjective quality measures and methods to increase the perceived quality by local QP adaptation are presented and discussed.
Keywords
Multimedia standards; Software; Application systems; Quality assessment and metrics
Instructors
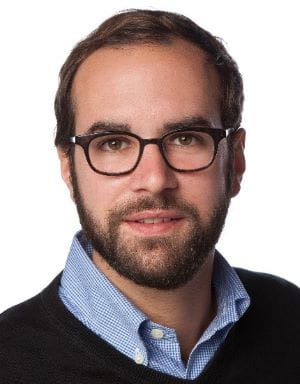
Benjamin Bross , Fraunhofer Heinrich Hertz Institute, Germany. (benjamin.bross [AT] hhi.fraunhofer.de)
Benjamin Bross received the Dipl.-Ing. degree in electrical engineering from RWTH Aachen University, Aachen, Germany, in 2008. In 2009, he joined the Fraunhofer Institute for Telecommunications – Heinrich Hertz Institute, Berlin, Germany, where he is currently heading the Video Coding Systems group at the Video Coding & Analytics Department, Berlin and a part-time lecturer at the HTW University of Applied Sciences Berlin. Since 2010, Benjamin is very actively involved in the ITU-T VCEG | ISO/IEC MPEG video coding standardization processes as a technical contributor, coordinator of core experiments and chief editor of the High Efficiency Video Coding (HEVC) standard [ITU-T H.265 | ISO/IEC 23008-2] and the emerging Versatile Video Coding (VVC) standard. In addition to his involvement in standardization, Benjamin is coordinating standard-compliant software implementation activities. This included the development of an HEVC encoder that is currently deployed in broadcast for HD and UHD TV channels as well as the optimized and open VVenC / VVdeC software implementations of VVC. Besides giving talks about recent video coding technologies, Benjamin Bross is an author or co-author of several fundamental HEVC and VVC-related publications, and an author of two book chapters on HEVC and Inter-Picture Prediction Techniques in HEVC. He received the IEEE Best Paper Award at the 2013 IEEE International Conference on Consumer Electronics – Berlin in 2013, the SMPTE Journal Certificate of Merit in 2014 and an Emmy Award at the 69th Engineering Emmy Awards in 2017 as part of the Joint Collaborative Team on Video Coding for its development of HEVC.
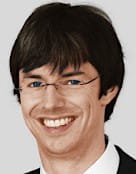
Christian Helmrich , Fraunhofer Heinrich Hertz Institute, Germany. (christian.helmrich [AT] hhi.fraunhofer.de)
Christian R. Helmrich received the B. Sc. degree in Computer Science from Capitol Technology University (formerly Capitol College), Laurel, MD in 2005 and the M. Sc. degree in Information and Media Technologies from the Technical University of Hamburg-Harburg, Germany, in 2008. Between 2008 and 2013 he worked on numerous speech and audio coding solutions at the Fraunhofer Institute for Integrated Circuits (IIS), Erlangen, Germany, partly as a Senior Engineer. From 2013 until 2016 Mr. Helmrich continued his work as a research assistant at the International Audio Laboratories Erlangen, a joint institution of Fraunhofer IIS and the University of Erlangen-Nuremberg, Germany, where he completed his Dr.-Ing. degree with a dissertation on audio signal analysis and coding. In 2016 Dr. Helmrich joined the Video Coding & Analytics Department of the Fraunhofer Heinrich Hertz Institute, Berlin, Germany, as a next-generation video coding researcher and developer. His main interests include audio and video acquisition, coding, storage, and preservation as well as restoration from analog sources. Dr. Helmrich participated in and contributed to several standardization activities in the fields of audio, speech, and video coding, most recently the 3GPP EVS standard (3GPP TS 26.441), the MPEG-H 3D Audio standard (ISO/IEC 23008-3), and the MPEG-I/ITU-T VVC standard (ISO/IEC 23090-3 and ITU-T H.266). He is author or co-author of more than 40 scientific publications and a Senior Member of the IEEE.
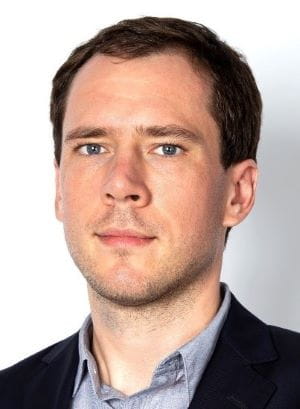
Adam Wieckowski , Fraunhofer Heinrich Hertz Institute, Germany. (adam.wieckowski [AT] hhi.fraunhofer.de)
Adam Wieckowski received the M.Sc. degree in computer engineering from the Technical University of Berlin, Berlin, Germany, in 2014. In 2016, he joined the Fraunhofer Institute for Telecommunications, Heinrich Hertz Institute, Berlin, as a Research Assistant. He worked on the development of the software, which later became the test model for VVC development. He contributed several technical contributions during the standardization of VVC. Since 2019, he has been a Project Manager coordinating the technical development of VVdeC and VVenC decoder and encoder solutions for the VVC standard.
Abstract
In the era of deep learning, neural architecture design plays a critical role in visual learning. For a variety of computer vision tasks, e.g., image recognition, semantic segmentation, object detection and action recognition, the backbone model is the workhorse for representation learning. In the past few years, many novel network architectures and associated design methodologies have been proposed. In this tutorial, we first introduce preliminaries and background of visual backbone network design. Then we present in-depth analysis of recent advances in this research field. Specifically, the tutorial will cover 1) the recent trend of applying Transformers, a highly successful originates from the field of NLP, to visual learning tasks; 2) neural architecture search, which is an automated network design approach; and 3) dynamic neural networks, which is a substitute of the widely used static deep learning models, for more efficient learning on edge devices.
Keywords
Deep learning for multimedia
Instructors
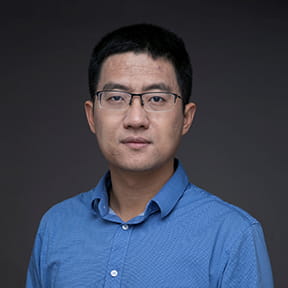
Jifeng Dai , SenseTime Research, China. (daijifeng [AT] sensetime.com)
Dr. Jifeng Dai is an Executive Research Director at SenseTime Research. He received both his B.S. and Ph.D. degrees from the Tsinghua University, in 2009 and 2014, respectively. He was a Visiting Scholar at the VCLA lab of UCLA, from 2012 to 2013. He worked at the Visual Computing Group of Microsoft Research Asia between 2014 and 2019. His current research interests are at the intersections of object recognition and deep learning. He authored about 30 papers on top-tier conferences and journals, and have collected more than 10,000 citations.
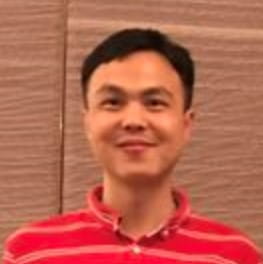
Xinggang Wang , Huazhong University of Science and Technology, China. (xgwang [AT] hust.edu.cn)
Dr. Xinggang Wang is an Associate Professor with the School of Electronic Information and Communications, Huazhong University of Science and Technology (HUST). He received the B.S. and Ph.D. degrees in electronics and information engineering from HUST in 2009 and 2014, respectively. He was a Visiting Scholar with UCLA and Temple University. His research interests are computer vision and machine learning, especially, data/label- and computation-efficient deep learning for visual recognition. He authored about 60 papers on top-tier conferences and journals, and have collected more than 5,400 citations. He serves as an Associate Editor for Image and Vision Computing.
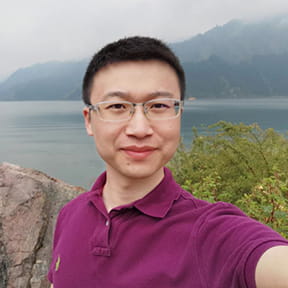
Gao Huang , Tsinghua University, China. (gaohuang [AT] tsinghua.edu.cn)
Dr. Gao Huang is an Assistant Professor in the Department of Automation at Tsinghua University. He received the Ph.D. degree from the Tsinghua University, in 2015. He was a Post-Doctoral Researcher with the Department of Computer Science, Cornell University, Ithaca, NY, USA, from 2015 to 2018. His current research interests include machine learning, computer vision, deep learning, and reinforcement learning. He authored about 50 papers on top-tier conferences and journals, and have collected more than 18,000 citations. His work on DenseNet won the Best Paper Award of CVPR (2017).
Abstract
Deep learning has achieved significant success in multimedia fields involving computer vision, natural language processing, and acoustics. However research in adversarial learning also shows that they are highly vulnerable to adversarial examples. Extensive works have demonstrated that adversarial examples could easily fool deep neural networks to wrong predictions threatening practical deep learning applications in both digital and physical world. Though challenging, discovering and harnessing adversarial attacks is beneficial for diagnosing model blind-spots and further understanding as well as improving deep learning systems in practice.
This tutorial will first introduce the definition and the recent applications of adversarial examples. Then it will discuss the typical attack and defense methods in the digital and physical world. Related topics such as backdoor attack and data poisoning will also be covered. Finally, this tutorial will present the connections between the adversarial examples and model robustness, and conclude with some future work.
Instructors
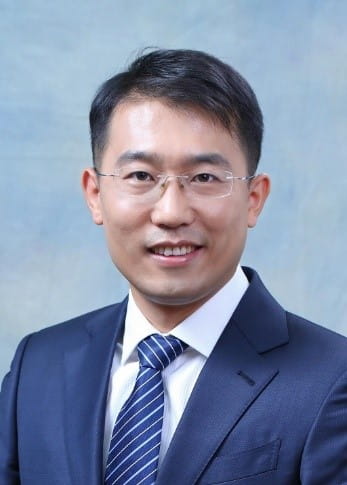
Xianglong Liu , Beihang University, China. (xlliu [AT] buaa.edu.cn)
Dr. Xianglong Liu is currently a full Professor with the School of Computer Science and Engineering, Beihang University. His research interests include fast visual computing (e.g., large-scale search/understanding) and trustworthy deep learning (e.g., network quantization, adversarial attack/defense, few shot learning). He authored more than 60 papers on top-tier conferences and journals. He is serving as the associated editor of Pattern Recognition, IET Image Processing, Frontiers of Computer Science, area chair of ACM MM 2019/2020/2021, etc. He is also the TPC member of the OpenI Open Source Platform for Artificial Intelligence. He received NSFC Excellent Young Scientists Fund, and was selected into the 2019 Beijing Nova Program and 2015 China Computer Federation (CCF) Young Talents Development Program. He also received a number of awards including PCM 2018 best student paper, IEEE ICME 2011 best paper candidate, IEEE CVPR 2014/2016 Young Researcher Award, the 2015 CCF Outstanding Doctoral Dissertation Award, etc.
Tutorial Chairs
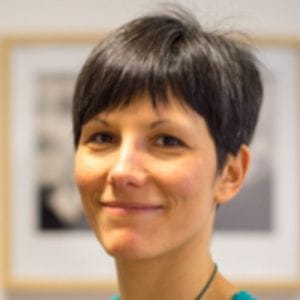
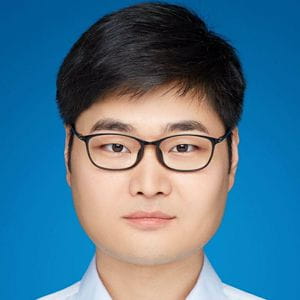