Special Sessions
Time
TBD
Room
TBD
Organizers
Yi Cai, South China University of Technology, China.
Zhenguo Yang, Guangdong University of Technology, China.
Abstract
Vision-and-Language research is an interesting area at the nexus of Computer Vision and Natural Language Processing, and has attracted rapidly growing attention from both communities. The general aims of holding this session is to provide a forum for reporting and discussing completed research that involves both language and vision and to enable NLP and computer vision researchers to meet, exchange ideas, expertise and technology, and form new research partnerships. Research involving both language and vision computing spans a variety of disciplines and applications, and goes back a number of decades. In a recent scene shift, the big data era has thrown up a multitude of tasks in which vision and language are inherently linked. The explosive growth of visual and textual data, both online and in private repositories by diverse institutions and companies, has led to urgent requirements in terms of search, processing and management of digital content. A variety of Vision and language tasks, benchmarked over large-scale human-annotated datasets, have driven tremendous progress in joint multimodal representation learning. This session will focus on some of the recently popular tasks in this domain such as visual captioning, visual grounding, visual question answering and reasoning, muti-modal dialogue, text-to-image generation, image-text retrieval, and multi-modal knowledge graph. We will invite or choose from this conference the most representative papers in these areas and discuss key principles that epitomize the core challenges & opportunities in multi-modal understanding, reasoning, and generation.
Important Dates (The same as the dates for regular papers)
-
Regular Paper submission (including Special Sessions) : November 29, 2020 - New Regular Paper Submission deadline: December 13, 2020 [11:59 p.m. PST]
- Regular Paper acceptance notification: TBD
- Camera-Ready Regular Paper submission deadline: TBD
Paper Submission
Authors should prepare their manuscript according to the Guide for Authors of ICME available at https://2021.ieeeicme.org/author_info.Time
TBD
Room
TBD
Organizers
Prof. Lin Li, Wuhan University of Technology, China.
Prof. Xi Shao, Nanjing University of Posts and Telecommunications, China.
Assistant Prof. Zheng Wang, The University of Tokyo, Japan.
Assistant Prof. Rajiv Ratn Shah, IIIT-Delhi, India
Prof. Yang Wang, Hefei University of Technology, China.
Website
https://wut-idea.github.io/ICME2021SS.github.io/Abstract
With the aim of provision of high-quality information, services and items to users, advanced Internet and multimedia services have experienced an exponential growth and advanced rapidly over the past decades. A huge amount of user-generated and service provider-generated multimedia data become available. These data are heterogeneous and multi-modal in nature, imposing great challenges for processing and analyzing them. Multi-modal data consist of a mixture of various types of data from different modalities such as texts, images, videos, audios etc. Data-driven correlational representation and knowledge-guided learning are the main scientific problems for multimedia analysis.
This special session aims at bringing together researchers and professionals from academia and industry from around the world for showcasing, discussing, and reviewing the whole spectrum of technological opportunities, challenges, solutions, and emerging applications in knoweldge-driven multi-modal deep analysis for multimedia. We especially encourage original work based on interdisciplinary research, such as computer science and social science, where quantitative evidence is available demonstrating the mutual advantage of such an approach.
Topics of particular interest include, but are not limited to:
- Multi-modal representation learning with knowledge
- Multi-modal data fusion with knowledge
- Knowledge representation for multi-modal data
- Deep cross-modality alignment with knowledge
- Methodology and architectures to improve model explainability with knowledge
- Multi-modal deep analysis for innovative multimedia applications, such as person reidentification, social network analysis, cross-modal retrieval, recommendation systems and so on.
Important Dates
-
Regular Paper submission (including Special Sessions) : November 29, 2020 - New Regular Paper Submission deadline: December 13, 2020 [11:59 p.m. PST]
- Submission of papers to workshops and other tracks : March 13, 2021
- Industry/Application track paper submission : March 22, 2021
Paper Submission
Authors should prepare their manuscript according to the Guide for Authors of ICME available at https://2021.ieeeicme.org/author_info. All papers presented at ICME 2021 will be included in IEEE Xplore. All papers submitted to this special session will go through the same review process as the regular papers submitted to the main conference to ensure that the contributions are of high quality. If our special session has more than 5 papers being accepted, some of the papers will be moved to the regular paper sessions of the conference.Time
TBD
Room
TBD
Organizers
Prof. Guangwei Gao (csggao [AT] gmail.com), Nangjing University of Posts and Telecommunications, China.
Prof. Junjun Jiang (jiangjunjun [AT] hit.edu.cn), Harbin Institute of Technology, China.
Prof. Licheng Liu (lichenghnu [AT] gmail.com), Hunan University, China.
Prof. Tao Lu (lutxyl [AT] gmail.com), Wuhan Institute of Technology, China.
Prof. Jingang Shi (jingang.shi [AT] hotmail.com), Xi'an Jiaotong University, China.
Website
https://guangweigao.github.io/icme21ss.html.Abstract
Representation learning has always been an important research area in pattern recognition. A good representation of practical data is critical to achieve satisfactory performance. Broadly speaking, such presentation can be “intra-data representation” or “inter-data representation”. Intra-data representation focuses on extracting or refining the raw feature of data point itself. Representative methods range from the early-staged hand-crafted feature design (e.g. SIFT, LBP, HoG, etc.), to the feature extraction (e.g. PCA, LDA, LLE, etc.) and feature selection (e.g. sparsity-based and submodulariry-based methods) in the past two decades, until the recent deep neural networks (e.g. CNN, RNN, etc.). Inter-data representation characterizes the relationship between different data points or the structure carried out by the dataset. For example, metric learning, kernel learning and causality reasoning investigate the spatial or temporal relationship among different examples, while subspace learning, manifold learning and clustering discover the underlying structural property inherited by the dataset.
Above analyses reflect that representation learning covers a wide range of research topics related to pattern recognition. On one hand, many new algorithms on representation learning are put forward every year to cater for the needs of processing and understanding various practical multimedia data. On the other hand, massive problems regarding representation learning still remain unsolved, especially for the big data and noisy data. Thereby, the objective of this special issue is to provide a stage for researchers all over the world to publish their latest and original results on representation learning. More details on our SS can be found at https://guangweigao.github.io/icme21ss.html.
Topics include but are not limited to:
- Metric learning and kernel learning
- Probabilistic Graphical Models
- Multi-view/Multi-modal learning
- Applications of representation learning
- Robust representation and coding
- Deep learning
- Domain transfer learning
- Unsupervised, semi-supervised, and supervised representation learning
Important Dates
-
Regular Paper submission (including Special Sessions) : November 29, 2020 - New Regular Paper Submission deadline: December 13, 2020 [11:59 p.m. PST]
- Submission of papers to workshops and other tracks : March 13, 2021
- Industry/Application track paper submission : March 22, 2021
Paper Submission
Authors should prepare their manuscript according to the Guide for Authors of ICME available at https://2021.ieeeicme.org/author_info.Time
TBD
Room
TBD
Organizers
Li Song (song_li [AT] sjtu.edu.cn), Professor, Shanghai Jiao Tong University, China.
Siwei Ma (swma [AT] pku.edu.cn), Professor, Peking University, China.
Dong Liu (dongeliu [AT] ustc.edu.cn), Associate Professor, University of Science and Technology of China, China.
Xin Zhao (xinzzhao [AT] tencent.com), Principal Researcher, Tencent America LLC, USA.
Jianjun Lei (jjlei [AT] tju.edu.cn), Professor, Tianjin University, China.
Abstract
New Generations of video coding techniques VVC, AVS3 and AV1 finalized their standard in July 2020, March 2019 and March 2018. Similar to the standards of previous generations such as HEVC, these new generations of standards still follow the typical hybrid video coding framework but includes more advanced coding tools at every module. These new coding tools help these standards save up to 50% bitrate over HEVC at the same perceptual quality. With the powerful feature representation and non-linear mapping ability, neural network-based video compressing methods outperformance HEVC a lot in previous pieces of literature. However, the potential of applying neural network to these new generations of standards is unclear. It is also curious whether neural network-based methods can still maintain superior performance. The significant bitrate saving of these new generations of standards is achieved at the cost of increased complexity. For example, it is reported that the encoding time of reference software VTM is 10x than HEVC under random access configuration. Therefore, at the current stage, it is necessary and of great value to explore algorithms to reduce the complexity of these standards while keeping the compression performance. On the other side, during the standardization, peak signal-to-noise ratio (PSNR) is still adopted as the objective quality metric to optimize. However, this pixel-wise objective metric correlates poorly with the human vision system (HVS). As the final video receiver, the HVS characteristics should be well excavated and utilized to achieve better perceptual quality. Consequently, this special session seeks submissions about the next generations of video coding techniques beyond VVC/AVS3/AV1. Topics of interest of this special session include, but are not limited to neural network-based new advanced coding technologies, complexity reduction algorithms and perceptual coding methods.
Paper Submission
Authors should prepare their manuscript according to the Guide for Authors of ICME available at https://2021.ieeeicme.org/author_info.Time
TBD
Room
TBD
Organizers
Mang Ye (mangye16 [AT] gmail.com), Research Scientist, Inception Institute of Artificial Intelligence, UAE.
Joey Tianyi Zhou (joey.tianyi.zhou [AT] gmail.com), Scientist, Agency for Science, Technology, and Research (A*STAR), Singapore.
Jianbing Shen (shenjianbingcg [AT] gmail.com), Full Professor, Beijing Institute of Technology, China.
Pong C. Yuen (pcyuen [AT] comp.hkbu.edu.hk), Chair Professor, Hong Kong Baptist University, Hong Kong, China.
Website
https://www.comp.hkbu.edu.hk/~mangye/ICME_SS.html.Abstract
Deep learning technique has been widely applied in multimedia analysis applications. With sufficient annotated training data, supervised deep learning has achieved inspiring performance on various vision tasks, such as image classification, face recognition, person re-identification. However, collecting enough annotated data needed for supervised methods requires extensive human annotation efforts. Considering that most of the learning performed by animals and humans is unsupervised, their predictive learning pattern provides a good guidance for unsupervised knowledge discovery from unlabeled data. To alleviate the reliance on human annotation, unsupervised/ weakly supervised learning has become an increasingly emergent topic in multimedia applications. However, it is still challenging for unsupervised/weakly supervised deep learning, as the raw image signal is in a continuous, high-dimensional space, and it also faces various obstacles, such as uncertain noise, large domain shift, heterogenous data.
The aim of this special issue is to call for a coordinated effort to investigate the deep learning techniques with limited supervision for different multimedia applications, identify key tasks and challenges, showcase innovative ideas, introduce large scale datasets for novel applications and discuss future directions. This special issue provides a forum for researchers from multimedia, computer vision, and machine learning to present recent progress in deep learning research with limited supervision to various multimedia applications. The list of possible topics includes, but not limited to:
- Unsupervised/ weakly-supervised learning for multimedia analysis
- Unsupervised domain adaptation for multimedia analysis
- Zero-shot learning for multimedia analysis
- Transfer learning for multimedia analysis
- Deep reinforcement learning with limited supervision for multimedia analysis
- Domain generalized learning for multimedia analysis
- Deep learning from web data with limited supervision
- New benchmarks/metrics/datasets for deep learning with limited supervision
Important Dates (the same with regular papers)
-
Regular Paper submission (including Special Sessions) : November 29, 2020 - New Regular Paper Submission deadline: December 13, 2020 [11:59 p.m. PST]
- Submission of papers to workshops and other tracks : March 13, 2021
- Industry/Application track paper submission : March 22, 2021
Paper Submission
Authors should prepare their manuscript according to the Guide for Authors of ICME available at https://2021.ieeeicme.org/author_info.Time
TBD
Room
TBD
Organizers
Changsheng Li (lcs [AT] bit.edu.cn), Professor, School of Computer Science and Technology, Beijing Institute of Technology, China.
Mingkui Tan (mingkuitan [AT] scut.edu.cn), Professor, School of Software Engineering, South China University of Technology, China.
Changqing Zhang (zhangchangqing [AT] tju.edu.cn), Associate Professor, College of Intelligence and Computing, Tianjin University, China.
Chang Tang (tangchang [AT] cug.edu.cn), Associate Professor, School of Computer Science, China University of Geosciences, China.
Abstract
Active learning techniques are designed for selecting informative or representative samples to be labeled, such that the costs of sample annotations are largely reduced while the prediction performance of the subsequent model can be guaranteed. Recent years have witnessed the rapid progress of deep neural networks on active learning for image and video tasks, including image classification, semantic segmentation, object tracking, action recognition, video retrieval, and so on. However, there is still a large room to design more effective learning mechanisms by leveraging deep learning to further boost the sample selection performance. Concurrently, it is also a valuable attempt to put existing research works into more industrial (or potential) applications. This special session seeks submissions about the latest deep learning based active learning models, methodologies, and applications. Topics of interest include, but are not limited to deep supervised active learning, deep unsupervised active learning, deep batch active learning, deep reinforcement active learning, deep self-paced active learning, and various applications of active learning.
Important Dates
-
Regular Paper submission (including Special Sessions) : November 29, 2020 - New Regular Paper Submission deadline: December 13, 2020 [11:59 p.m. PST]
Paper Submission
Authors should prepare their manuscript according to the Guide for Authors of ICME available at https://2021.ieeeicme.org/author_info.Time
TBD
Room
TBD
Organizers
Chang-Tsun Li, Deakin University, Australia.
Chin-Chen Chang, Feng-Jia University, Taiwan.
Li Li, Hangzhou Dianzi University, China.
Abstract
Multimedia carries not only the value of its content, but also its value in digital forensics for combating crimes and fraudulent activities. With its apparent commercial value, multimedia piracy continues to be an acute issue, especially in the entertainment industry, despite the earnest search for solutions in the past two decades. Deliberate manipulations of multimedia content for malicious purposes have also been a prevailing problem in many walks of life. With the phenomenal leap of artificial intelligence and machine learning (deep learning in particular) in recent years, realistically forged multimedia is appearing as disinformation and fake news that are impacting on social justice at the personal level and major political campaigns and national security at the national level, as well as affecting the stability of international relationships at the global level. In light of the afore-mentioned issues, this proposed special session is intended as a forum for fostering cross-fertilization of ideas that can be called upon to protect the value and integrity of multimedia content. As such, it is dedicated to disseminate recent advances in multimedia forensics and security technologies and their applications in the real world, in particular in the following research topics:
- Multimedia (including social media) provenance attribution and identification
- Fake multimedia detection
- Multimedia content integrity verification through intrinsic and extrinsic data
- Multimedia copyright protection through digital watermarking
- Industry applications of multimedia forensics and security
- Anti-forensics and counter anti-forensics measures
Important Dates
-
Regular Paper submission (including Special Sessions) : November 29, 2020 - New Regular Paper Submission deadline: December 13, 2020 [11:59 p.m. PST]
Paper Submission
Authors should prepare their manuscript according to the Guide for Authors of ICME available at https://2021.ieeeicme.org/author_info.Time
TBD
Room
TBD
Organizers
Yao Zhao (yzhao [AT] bjtu.edu.cn), Professor, Beijing Jiaotong University, China.
Runmin Cong (rmcong [AT] bjtu.edu.cn), Associate Professor, Beijing Jiaotong University, China.
Sam Kwong (cssamk [AT] cityu.edu.hk), Chair Professor, City University of Hong Kong, China.
Chunjie Zhang (cjzhang [AT] bjtu.edu.cn), Professor, Beijing Jiaotong University, China.
Huihui Bai (hhbai [AT] bjtu.edu.cn), Professor, Beijing Jiaotong University, China.
Hui Yuan (huiyuan [AT] sdu.edu.cn), Professor, Shandong University, China.
Abstract
Biologically, the human visual system can not only perceive appearance information such as the color and texture, but also capture depth information of the scene through the binocular structure and generate stereo perception. Inspired by the human stereo perception system, depth sensing has become one of core components of many computer vision tasks, such as 3D scene reconstruction, virtual reality, scene understanding, and automatic drive. With the rapid development of depth imaging technologies and hardware devices, the acquisition of depth information has become more convenient and even some smartphones are equipped with depth sensors. Although depth information enables machines to comprehensively understand the objective world, it also brings some new problems and challenges in depth-related processing and applications. We invite full-length papers related (but not limited) to the following topics for depth processing together with widespread applications.
- Depth related processing, such as
- depth estimation from single image/stereo image/light field image/video
- depth map enhancement, including hole-filling, denoising, completion, super-resolution
- depth/disparity adjustment and registration
- depth map/video coding and compression
- point cloud data processing, including upsampling, detection, segmentation
- Depth assisted applications, such as
- low-level applications, including editing, inpainting, retargeting, matching, quality assessment, etc
- high-level applications, including segmentation, recognition, classification, object detection, tracking, reconstruction, retrieval, SLAM, pose estimation, scene understanding, etc
Important Dates
-
Regular Paper submission (including Special Sessions) : November 29, 2020 - New Regular Paper Submission deadline: December 13, 2020 [11:59 p.m. PST]
- Submission of papers to workshops and other tracks : March 13, 2021
- Industry/Application track paper submission : March 22, 2021
Paper Submission
Authors should prepare their manuscript according to the Guide for Authors of ICME available at https://2021.ieeeicme.org/author_info.Time
TBD
Room
TBD
Organizers
Minh-Son Dao (dao [AT] nict.go.jp), Senior researcher, National Institute of Information and Communications Technology (NICT) Japan.
Cathal Gurrin (cathal.gurrin [AT] dcu.ie), Associate professor, Dublin City University (DCU), Ireland.
Duc-Tien Dang-Nguyen (ductien.dangnguyen [AT] uib.no), Associate professor, University of Bergen (UiB), Norway.
Thanh-Binh Nguyen (ngtbinh [AT] hcmus.edu.vn), Director of the AISIA Research Lab, Vietnam National University in HCM city (VNU-HCMUS).
Abstract
The association between people's wellbeing and the surrounding environment's properties is a vital area of investigation. Although these investigations have a long and rich history, they have focused on the general population. There is a surprising lack of research investigating the impact of the environment on individual people's scale where local information about air pollution (e.g., PM2.5, NO2, O3), weather (e.g., temperature, humidity), urban nature (e.g., greenness, liveliness, quietness), multimedia data (e.g., street view images, satellite images, CCTV, personal camera data), and individual behavior (e.g., psychophysiological data, SNS data) play an essential role. It is not always possible to gather plentiful amounts of such cross-data. As a result, a key research question remains open: Can sparse or incomplete data be used to gain insight into wellbeing? Is there a hypothesis about the data's associations so that wellbeing can be understood using a limited amount of data from usual and personal devices? How about the privacy of people when sharing their information? Developing hypotheses about the associations within the heterogeneous data contributes to building good multimodal models that make it possible to understand the impact of the environment on wellbeing at the local and individual scale. Such models are necessary since (1) not all cities are fully covered by the standard and high-end technology stations (e,g, air pollution, and weather stations, CCTV system, satellite connections); (2) not all people can access data generated by these resources; and (3) not all people experience the same reaction to the same environmental situation. Besides, a system that aims to collect and analyze data containing individuals' sensitive information needs to be secured to protect privacy.
This special session seeks innovative papers that exploit novel technologies and solutions from both industry and academia on understanding the surrounding environment's impact on human lives, especially on a local and individual scale. This special session also targets (but is not limited to) researchers in multimedia information retrieval, machine learning, AI, data science, event-based processing and analysis, multimodal content analysis, lifelog data analysis, IoT, security, healthcare, urban computing, environmental science, and atmospheric science.
Important Dates
-
Regular Paper submission (including Special Sessions) : November 29, 2020 - New Regular Paper Submission deadline: December 13, 2020 [11:59 p.m. PST]
Paper Submission
Authors should prepare their manuscript according to the Guide for Authors of ICME available at https://2021.ieeeicme.org/author_info.Special Session Chairs
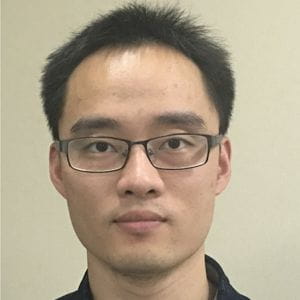
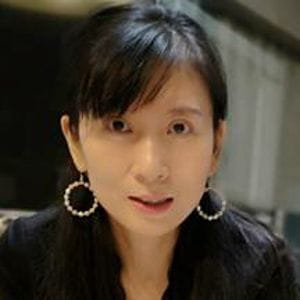