Demonstrations
Time
TBD
Authors
Qian Liu (Dalian University of Technology); Shuang Wang (Dalian University of Technology); Bing Wu (Dalian University of Technology)
Abstract
In this paper, we present a flexible 3D haptic tactile sensor array which can be utilized to build up the foundation of haptic rendering in multimodal human-machine interaction applications, e.g. virtual reality (VR) games. It is known that current commercialized haptic VR devices are all actuators. They require the raw sensing data of human touch operations to develop sophisticated haptic rendering algorithms in order to mimic the sense of human haptic feedback. To this end, we designed a novel flexible, distributed sensor array and the corresponding force signal detection kit. The proposed haptic sensor consists of three sides (i.e. front, left, right) and can be applied to human fingertips in order to capture the 3D force feedback of human grasping. This is in strong contrast to the current haptic sensors which can generally capture the normal pressure (at the front side) alone. Experimental results show that the proposed sensor has a detection precision of 0.1N in the range of 0 to 20N, and a response time of 23.8ms.
Time
TBD
Authors
Delong Chen (Hohai University); Fan Liu (Hohai University); Feng Xu (Hohai University)
Abstract
In this demo, we present VirtualConductor, a system that can generate conducting video from any given music and a single user's image. First, a large-scale conductor motion dataset is collected and constructed. Then, we propose Audio Motion Correspondence Network (AMCNet) and adversarial-perceptual learning to learn the cross-modal relationship and generate diverse, plausible, music-synchronized motion. Finally, we combine 3D animation rendering and a pose transfer model to synthesize conducting video from a single given user's image. Therefore, any user can become a virtual conductor through the system.
Time
TBD
Authors
Fei Gao (Hangzhou Dianzi University); Jingjie Zhu (Hangzhou Dianzi University); Weiyu Weng (Hangzhou Dianzi University); Chenyang Jiang (AiSketcher Technology); Xiang Li (Hangzhou Dianzi University); Xiao Zhu (AiSketcher Technology); Lingna Dai (Hangzhou Dianzi University); Peng Li (Peking University)
Abstract
In this work, we develop algorithms which can translate a facial photo to three styles of artistic portrait drawings, including line-, pen-, and pencil-drawings. Afterwards, we develop three demonstrations, including an applet of WeChat, a Web API, and a drawing robot. By using the former two demonstrations, one can upload a facial photo, choose an artistic style, and then obtain the corresponding portrait drawing in seconds. Our drawing robot can finish a line-drawing portrait on paper in about two minutes, after a user scans a QR code and upload a facial photo. Our demonstrations have attended a quite number of exhibitions and shown remarkable performance under diverse circumstances. We have made the core of our work (paper 1551 in the main track) publicly available: http://aiart.live/genre/.
Time
TBD
Authors
Zhibin Chen (Hohai University); Fan Liu (Hohai University); Delong Chen (Hohai University); Jingyu He (Hohai University)
Abstract
In traditional cognitive diagnosis models, the representations of students and questions tend to have a high correlation. It results in biases and poor performance in real-world applications. In order to weaken such correlation, we propose a Weakly Correlated Adversarial Learning (WCAL) method. Based on WCAL, we design a cognitive system for both student knowledge state evaluation and exam results prediction which can help teachers select exams suitable for students. The experimental results show the proposed method can effectively model students’ knowledge state and help teachers improve the teaching effect.
Demo Chairs
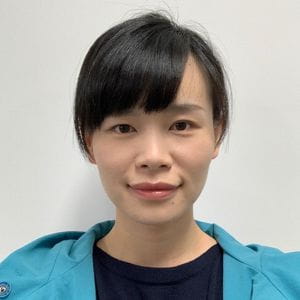
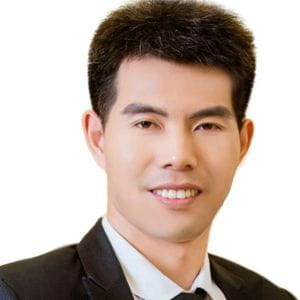