Multimedia Rising Star
Overview
The Multimedia Rising Star Program was initiated in ICME 2021 to highlight plenary presentations of about four selected rising researchers on their vision and research achievements, and dialogs with senior members about the future of multimedia research. The Multimedia Rising Star Award will be selected every two years at ICME conferences, aiming to acknowledge young and accomplished researchers working in emerging areas of multimedia research.
Nomination Process
The nominations of rising star candidates are made from the four sponsoring TCs. Each TC can nominate no more than 3 candidates from the TC members. The candidates are expected to graduate with their PhD within 10 years. We encourage TCs to consider diversity, including regional and gender balance when selecting the candidates. Based on the inputs from TCs, a committee including the ICME 2021 Panel Co-chairs (Gary Li, Marta Mrak, Shervin Shirmohammadi) will select the final rising stars. In this process, multiple factors will be carefully considered, including created and expected impact (both academic and non-academic) of their work, scholastic and industrial achievements after their PhD degree, professional service records, and proposed presentation topics of nominated candidates. Other relevant achievements should also be highlighted in the nomination documentation for the candidates.
Evaluation and Election Process
Up to four rising stars selected by the panel chairs will be invited by ICME 2021 to join a forum and deliver a talk during the conference, with their registrations waived. An award committee will be formed to review the nomination materials, and elect the Multimedia Rising Star from the four final candidates. A certificate will be presented to each selected Multimedia Rising Star by ICME 2021 Organizing Committee during the Award Ceremony.
Important Dates
-
May 15, candidates recommended from 4 sponsoring TCs. TC chairs to send recommendations together with (1) candidates’ CVs, and (2) support letter addressing selection criteria, including candidates’ talk titles and abstracts, to Gary Li (geli@pku.edu.cn), Marta Mrak (marta.mrak@bbc.co.uk), and Shervin Shirmohammadi (shervin@eecs.uottawa.ca).
-
May 30, selection of finalist by the committee.
-
July 6, multimedia rising star panel.
-
July 8, delivery of Multimedia Rising Star Award at the closing ceremony of ICME 2021.
Multimedia Rising Star Candidates
Title
Efficient Compression, Processing and Analysis for 3D Immersive Media
Abstract
With the significant progresses of recent 3D technologies (particularly in point cloud, light field and RGB-D, etc.), immersive media has become more and more popular and attracted much attention. Efficient compression, processing and analysis are urgently required for providing better visual experience and empower the related machine vision systems, such as the emerging applications in panoramic movies and autonomous driving, etc. In this talk, we will give several exemplary solutions for compression, restoration and analysis. First, for compression issue, a novel layer-wise geometry aggregation (LGA) framework is investigated for LiDAR point cloud lossless geometry compression, where “divide-and-conquer” scheme is effectively devised to deal with the diversified point content. Second, for restoration issue, we have also developed a biology-inspired vaccine-style-net for point cloud completion and a reinforcement learning method for quality enhancement of single and mixed distorted images. Third, for analysis issue, we also present a unified information fusion network for efficient RGB-D and RGB-T salient object detection (possibly including light field or point cloud analysis). Finally, the future works will be discussed. From this talk, we would like to inspire the interests and attention from audiences in developing more effective learning paradigms and analytical models for better performances on compression, processing and analysis of the emerging 3D immersive media, and expedite the related technology evolution.
Instructors
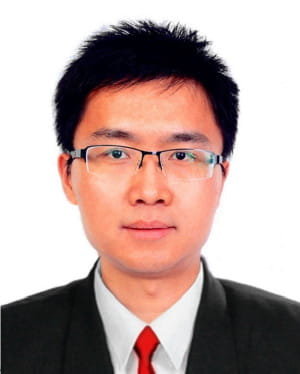
Wei Gao , School of Electronic and Computer Engineering, Shenzhen Graduate School, Peking University, China.
Dr. Wei Gao is currently an Assistant Professor with the School of Electronic and Computer Engineering, Peking University (PKU), and also affiliated to Peng Cheng Laboratory, Shenzhen, China. He is now leading the Immersive Video Perception (IVP) Group at PKU. He received the Ph.D. degree in Computer Science with the Department of Computer Science, City University of Hong Kong (CityU), Kowloon, Hong Kong, in February 2017. In 2016, he was a Visiting Scholar with University of California, Los Angeles (UCLA), CA, USA. From 2017 to 2019, he was a Postdoctoral Fellow with City University of Hong Kong, Hong Kong, and Research Fellow with Nanyang Technological University, Singapore. From 2012 to 2013, he was a Camera ISP Engineer with the OmniVision Technologies, Shanghai, China, where several completed high performance camera ISP and CMOS Image Sensor have been successfully taped out. His recent research works have been published in high quality journals and conferences, and applied over 30+ US and China patents. He has won two outstanding paper awards as the first author, and is also on the editorial board of three international journals in the field of multimedia computing and machine learning. His current research mainly focuses on the effective compression and processing algorithms and systems for 3D immersive media.
Title
Interpretable Graph Spectral Processing and Analysis of Geometric Data
Abstract
Geometric data acquired from real-world scenes, e.g., 2D depth images, 3D point clouds, and 4D dynamic point clouds, have found a wide range of applications including autonomous driving, augmented and virtual reality, surveillance, etc. Due to irregular sampling patterns of most geometric data, traditional image/video processing methodologies are limited, while Graph Signal Processing (GSP)—a fast-developing field in the signal processing community—enables processing signals that reside on irregular domains. Further, GSP provides insightful spectral interpretations and domain knowledge for the recently developed Graph Neural Networks (GNNs), leading to interpretability and robustness of GNNs. In this talk, I will describe three on-going research projects to illustrate the power of graph spectral processing and analysis. The first project studies spectral graph learning for point cloud denoising when the number of signal observations is extremely small—just one observation or even fewer. The second project on point cloud segmentation introduces domain knowledge via GSP-based regularization, which essentially enforces the features of vertices within each connected component of the graph to be similar and thus enables interpretable and robust segmentation. Finally, in the third project, we propose an unsupervised learning of Graph Transformation Equivariant Representations (GraphTER), aiming to capture intrinsic patterns of graph structure under graph signal transformations inspired by GSP, which approaches the upper bound set by the fully supervised counterparts for point cloud classification and segmentation.
Instructors
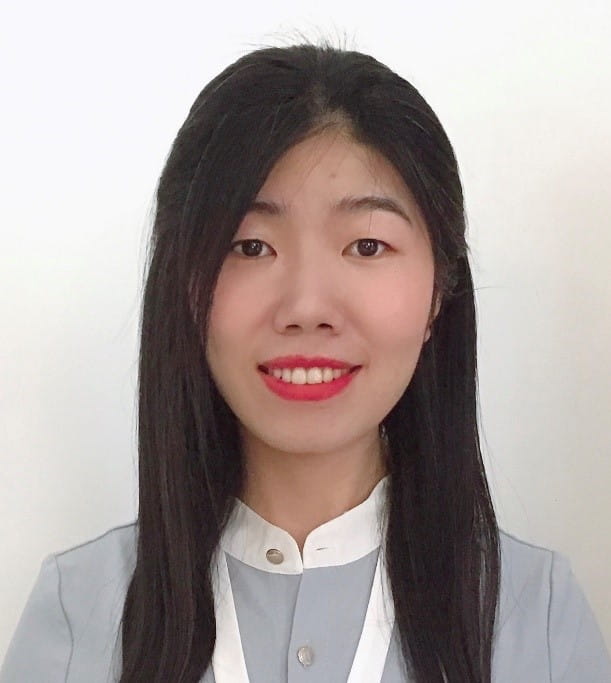
Wei Hu , Peking University, China.
Wei Hu received the B.S. degree in Electrical Engineering from the University of Science and Technology of China in 2010, and the Ph.D. degree in Electronic and Computer Engineering from the Hong Kong University of Science and Technology in 2015. She was a Researcher with Technicolor, Rennes, France, from 2015 to 2017. She is currently an Assistant Professor with Wangxuan Institute of Computer Technology, Peking University. Her research interests are graph signal processing, graph-based machine learning and 3D visual computing. Dr. Hu has authored around 50 international journal and conference publications, with several paper awards including the Best Student Paper Runner Up Award in ICME 2020 and Best Paper Candidate in CVPR 2021. She was awarded the Peking University Boya Young Fellow. She has been a member of IEEE MSA-TC and associate member of IEEE MMSP-TC, and an Associate Editor for Signal Processing Magazine, IEEE Transactions on Signal and Information Processing over Networks and Frontiers in Signal Processing. She served as the Open Source Chair of ICME 2021, and an Area Chair of ICME 2020 and ACM MM 2020. Also, she co-organized special sessions in ICME 2020 and ICIP 2021, and a workshop in ICCV 2021.
Title
Progressive Search Paradigm for Open-world Instance Re-identification
Abstract
The vast array of multimedia sensing technologies have produced a huge variety of big multi-modal data. The open-world instance re-identification (re-ID), whose primary tasks are finding a specific person/vehicle/object of interest with the multimedia sensing data, has tremendous potential applications. However, the open-world instance re-ID still faces the challenges of the guarantee of computing timeliness, the complex and instantly changed physical environments, the correlation discovery of massive multiple-modality data, and information security and privacy protection. To solve the challenges, we present a progressive search paradigm, which contains three important search strategies: 1) coarse-to-fine search in feature space; 2) near-to-distant search in spatial-temporal space; and 3) low-to-high permission search in the security space. The strategies all utilize simple features and computation to instantly reduce the search space, in which a complex matching process can be efficiently exploited to find the matched objects finely. Finally, we present our real-world produces implemented in the multimedia sensing network, which demonstrates the proposed progressive search paradigm can significantly improve the open-world Instance re-ID speed and accuracy.
Instructors
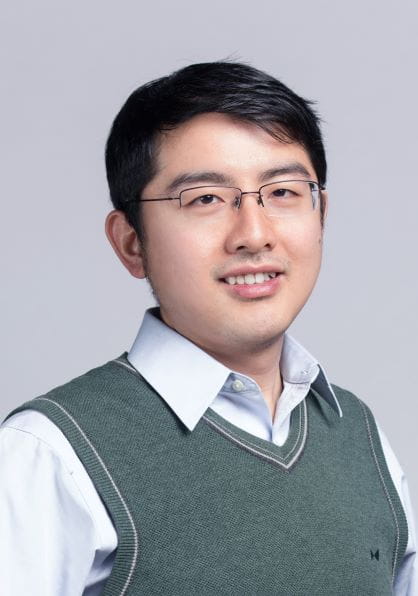
Wu Liu , JD AI Research, China.
Dr. Wu Liu is a Senior Researcher in JD AI Research, China. His current research interests include human behavior analysis and intelligent video surveillance. He received his Ph.D. degree from the Institute of Computing Technology, Chinese Academy of Science in 2015. He has published more than 80 papers in prestigious conferences and journals in computer vision and multimedia. He received IEEE Trans. on Multimedia 2019 Prize Paper Award, IEEE Multimedia 2018 Best Paper Award, IEEE ICME 2016 Best Student Paper Award, 2021 MSA-TC Best Paper Award-Honorable Mention, and Chinese Academy of Sciences Outstanding Ph.D. Thesis Award in 2016, etc. He also won the 1st Place in the single and multi-person pose estimation tasks in CVPR 2018 Look into Person Challenge, and the 2020 National Artificial Intelligence Competition for Rerson Reidentification. Dr. Liu is the founding committee member of ACM FCA, and the committee member of IEEE CASS-MSA. He has also served as the Technical Program Chair of ACM MM Asia 2021, Web Chair of ICME 2019, Publicity Chair of BIGMM 2018, Industrial Chairs of ChinaMM 2020&2021, and the Area Chairs of ACM MM 2019-2021, AAAI 2021, ICME 2019, ICIP 2017, etc. Wu Liu also organized the three workshops in ACM MM 2021&2020 and IEEE ICCV 2021, three tutorials in ACM MM 2020, IEEE ICME 2019 and ACM MM Asia 2019, three special issues in IEEE TCSVT 2021, MVA 2018, and MTAP 2019. Wu Liu also won the IEEE ICME 2019 Outstanding Service Awards.
Title
Compact Video Representation Towards Machine Perception
Abstract
The ultimate receiver of video data has recently experienced a radical shift from traditional human vision to machine vision, due to an unprecedented booming of visual intelligence-based applications fueled by the advances of artificial intelligence. In this context, the compact representation
of video data is of prominent importance, as there is essentially no value if the continuously acquired visual data could not be efficiently represented. In this presentation, I will introduce our recent works on compact video representation towards machine perception. In particular, the visual data compression algorithms are developed at both signal-level and feature level, enjoying the advantages of promising efficiency, enhanced flexibility as well as guaranteed security. The quality evaluation model, which is designed from the perspective of ultimate utility, could further serve as the optimization goal based upon the unified measure from multiple objectives. Finally, I will summarize our activities regarding the standardization of the compact video representation for machine vision, and envision the future research towards seamless and unified visual information representation.
Instructors
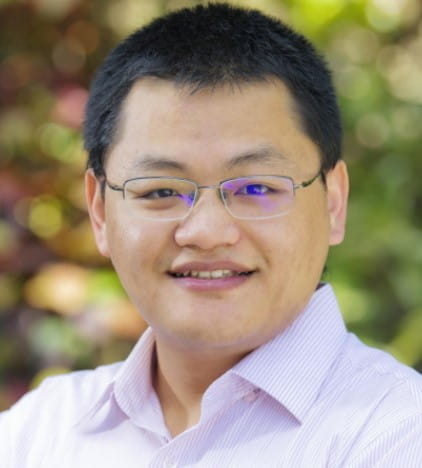
Shiqi Wang , City University of Hong Kong, China.
Shiqi Wang is an Assistant Professor of Computer Science at City University of Hong Kong. He holds a Ph.D. (2014) from the Peking University and a B.Sc. in Computer Science from the Harbin Institute of Technology (2008). From Mar. 2014 to Mar. 2016, he was a Postdoc Fellow with the Department of Electrical and Computer Engineering, University of Waterloo, Waterloo, Canada. From Apr. 2016 to Apr. 2017, He was with the Rapid-Rich Object Search Laboratory, Nanyang Technological University, Singapore, as a Research Fellow. His primary research interests include image and video coding, processing and analytics. He has proposed over 50 technical proposals to ISO/MPEG, ITU-T and AVS standards, and authored/co-authored more than 200 refereed journal/conference papers, including more than 70 IEEE transactions. He received the NSFC Excellent Young Scientist Fund (HK & Macau) 2020, Best Paper Award of IEEE ICME 2019, IEEE VCIP 2019, IEEE Multimedia 2018, PCM 2017, and is the co-author of a paper that received the Best Student Paper Award in IEEE ICIP 2018.
Multimedia Rising Star Competition Organisers
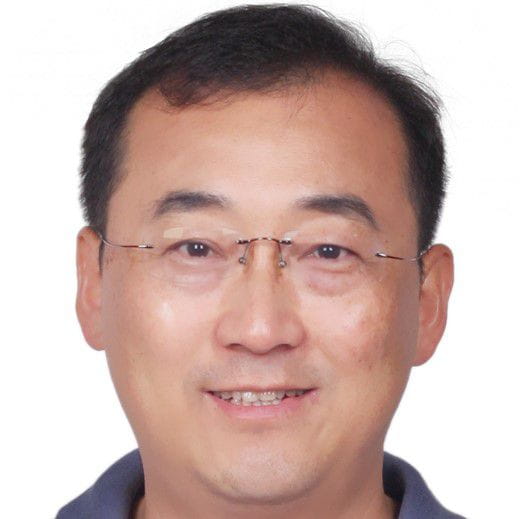
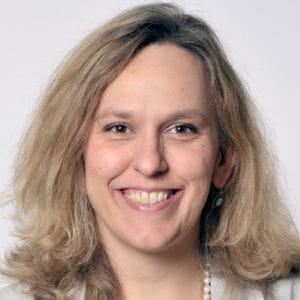
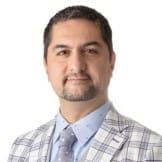
Multimedia Rising Star Committee
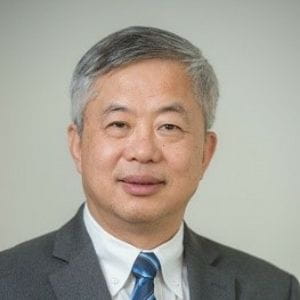
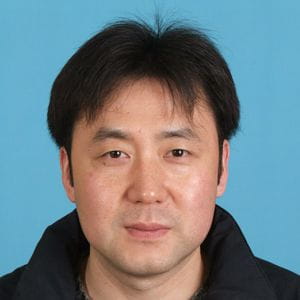
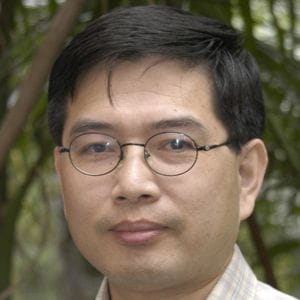
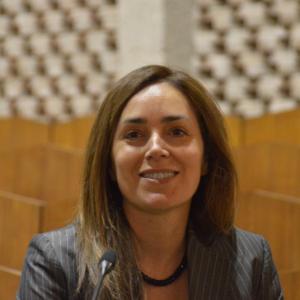
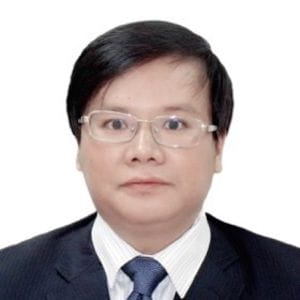
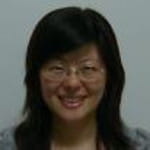
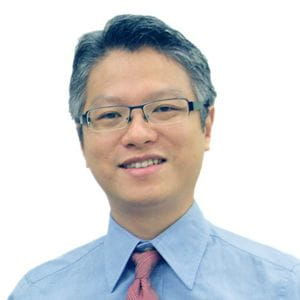
Industry Sponsor
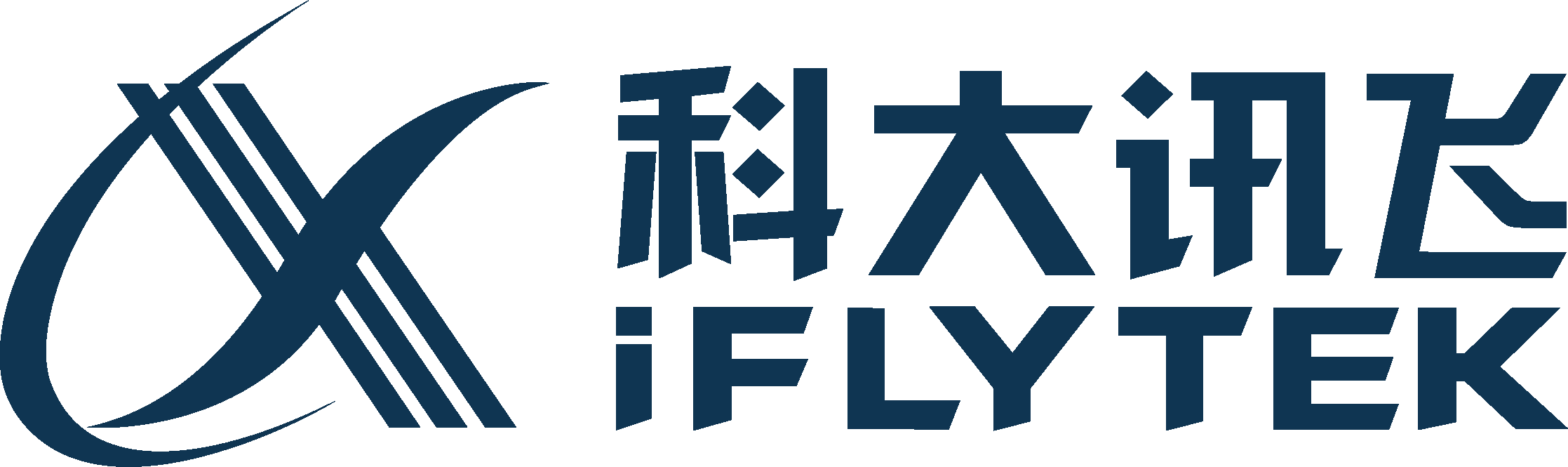