Grand Challenges
Time
TBD
Room
TBD
Organizers
Ying Liu, Xi'an University of Posts and Telecommunications.
Da Ai, Xi’an University of Posts and Telecommunications.
Wei Zhang, JD AI Research.
Website
http://www.xuptciip.com.cn/show.html?news-mgc2021Description
Background: Tire pattern recognition is an important means in providing clues for traffic accident management. With the rapid increase in the number of vehicles in use, there is urgent need to develop efficient and automatic vehicle tire recognition system. With limited amount of training samples available, accurate tire pattern recognition is a challenging task.
Data: Over 10,000 tire pattern images, in 69 classes. Each class contains tire surface pattern images and indentation mark images taken under different conditions, at different scales and different angles.
Task: Design a few-shot learning model for tire pattern image classification and retrieval. The model should provide satisfactory performance on tire pattern recognition(classification), tested on tire tread patterns, or indentation marks, or the mix of both. Given query image as either tread pattern or tire indentation mark, the algorithm should also provide high precision in tire pattern retrieval, finding the tire surface and tire indentation mark images of same tire model. In addition, the tire feature obtained must be robust so as to give reasonably good performance when tested on on-site tire pattern images which are of low quality.
Time
TBD
Room
TBD
Organizers
Haiqiang Wang, Peng Cheng Lab.
Gary Li, Peking University.
Shan Liu, Media lab, Tencent.
C.-C. Jay Kuo, University of Southern California.
Website
http://ugcvqa.com/Description
Video Quality Assessment (VQA) has been an active research field in both academic and industry in the last two decades. Recently, the growing popularity of video sharing applications and video conferencing systems is posing new challenges to the VQA field. Indeed, User Generated Content (UGC) videos in these applications exhibit quite different characteristics than Professionally Generated Content (PGC) videos. UGC videos are commonly captured by amateurs using smartphone cameras under various shooting conditions. The captured videos are often processed with special effects and aesthetics filters before being compressed and uploaded to video sharing applications.
With the assumption that pristine PGC videos possess perfect quality, FR VQA metrics predict the quality of processed videos by measuring quality degradation between reference and processed videos. However, this assumption is generally not valid for UGC videos and there is a need to develop new techniques to close the gap between PGC and UGC videos.
This challenge is focused on estimating the quality of H.264/AVC compressed UGC videos. There are two tracks depends on whether any information of the reference is used:
- The MOS track, an algorithm would predict the Mean Opinion Score (MOS) of compressed clips. Please note that the test set includes both the "references" and their compressed versions in this track.
- The DMOS track, the Differential Mean Opinion Score (DMOS) between the reference and compressed clips should be predicted.
Time
TBD
Room
TBD
Organizers
Yinglu Liu, JDTech, JD.com.
Mingcan Xiang, JDTech, JD.com.
Hailin Shi, JDTech, JD.com.
Wu Liu, JDTech, JD.com.
Xiangyu Zhu, Institute of Automation, Chinese Academy of Sciences.
Website
https://fllc3-icme2021.github.io/Description
Due to the global pandemic of COVID-19, people are recommended to wear facial masks for health and safety reasons, and the situation will continue in the long term. This apparently makes conventional facial landmark localization unfaithful and inefficient. However, facial landmark localization is a very crucial step of facial recognition technology, which is very helpful in tracking the close contacts of COVID-19 patients to prevent the spread of the virus. Besides, it is also wildly used in head pose estimation, face image synthesis, etc. Therefore, we are hosting the 3rd grand challenge of 106-point facial landmark localization in conjunction with ICME 2021, aiming to improve the accuracy and efficiency of facial landmark localization in real-world situations, especially on masked faces.
The 1st and 2nd 106-point facial landmark localization competitions were held in conjunctive with ICME2019 and ICPR2020, respectively. There are more than 400 teams taking part in the competitions, e.g., Tinghua University, National University of Singapore, University of Michigan. Different from the prior two challenges, the 2021 edition contains about 27,000 images of three kinds, real-masked, virtual-masked, and non-masked, which are largely varied in identity, pose, expression, and occlusion. In addition, a strict limitation of model weights is required for computational efficiency (the upper bound of computational complexity is 100MFLOPs, and the upper bound of model size is 2MB). We sincerely invite academic and industrial practitioners to participate in and together push the frontier along this direction.
Grand Challenge Chairs
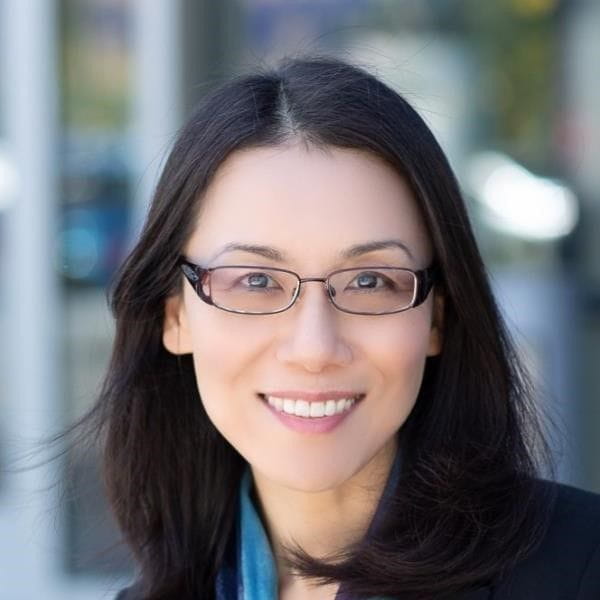
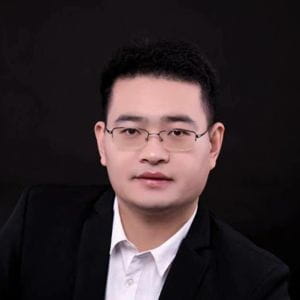
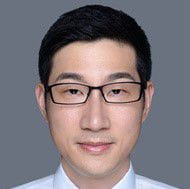